Summary
Recommendation engines use a combination of AI and ML technology. Hybrid, Collaborative filtering, and Content-based filtering are the most appreciated types of recommendation systems. Each of them can cater to the unique needs of business websites.
Main content
As pointed out by late Steve Jobs, “People don’t know what they want until you show it to them.” And this is what recommender systems do. Also referred to as a recommendation engine or algorithm, the program analyzes user behavior and recommends products that the individual may like. Put simply, the technology uses a combination of Artificial Intelligence and Machine Learning to explore a huge chunk of consumer data for making predictions.
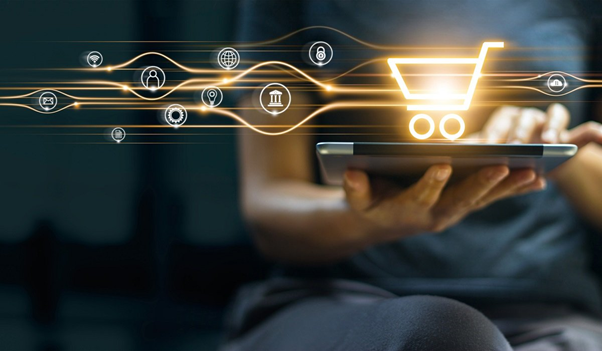
Here’s a detailed look at the exciting aspects surrounding recommendation engines:
- Popular types of recommendation systems in use
- Remarkable examples
- The math factor
- Best ways to implement this technology
Popular types of recommendation systems in use
Content-based filtering
The content-based filtering method involves offering recommendations based on features of items that the individual had chosen in the past along with the data associated with the user profile, including metadata.
Collaborative filtering
There is a thin line between collaborative and content-based filtering. Collaborative filtering involves analyzing user preferences, activities, and behaviors. Then, this data is compared with the behavior of other users for predicting what the particular individual may like. Put simply, consumer’s preferences are predicted based on what customers in a similar group choose. Amazon uses this filtering to offer recommendations.
Hybrid recommendation
Collaborative filtering comes with a set of problems, including a cold start. On the other hand, a content-based approach only focuses on existing interests. The hybrid approach proves to be more effective in some cases as it combines the techniques from collaborative and content-based strategies. In other words, it combines items popular among peers, products that fit the user’s needs, combined with things that the person liked in the past. Netflix uses this model. The streaming giant offers recommendations by combining movies with shared characteristics; films searched by users with similar habits, and the subscriber’s watch history.
Remarkable examples
As pointed earlier, Amazon uses a recommender algorithm that suggests products to buyers based on certain factors. Spotify generates a playlist of songs, while Netflix recommends movies to subscribers using an almost similar system. Likewise, LinkedIn uses a recommendation engine to recommend pages, newsletters, and even jobs.
The math factor
- A study published by Forrester research has pointed out that more than 15 percent of users end up buying products recommended on e-commerce sites.
- A McKinsey and Company study about the significance of personalization has highlighted product recommendations can result in a 5 to 15 percent increase in the business’s revenue.
- Roughly more than 75 percent of streaming on Netflix happens due to recommendation algorithms.
Points to be considered before implementing this technology
Specific recommendation engines follow user activities on the internet, while others suggest products based on past interactions and user location. You can also design and implement engines that recommend items as per predefined rules and manual inputs (keywords). Thus, implementing a recommendation engine needs a long-term strategy.
Besides e-commerce and media, even the telecom, retail, utilities, and banking sector companies can benefit from such systems. You can choose a software vendor for assistance in implementing pre-built recommendation engine apps or rest-based APIs on your site.
You can let a reputed IT firm that has handled clients from your industry work on your project. Such software vendors can assist you in selecting and deploying the best suitable recommendation engine on your business website.
Author Bio
Nitten Gokhaley is a content writer/journalist with nine years of experience in writing for news and digital marketing agencies. Despite being an arts graduate, he keenly follows tech news from around the world.